Technology
Jun 17, 2021
The End of Third-Party Cookies Part 3: Strengthening Post-Cookie Customer Ties
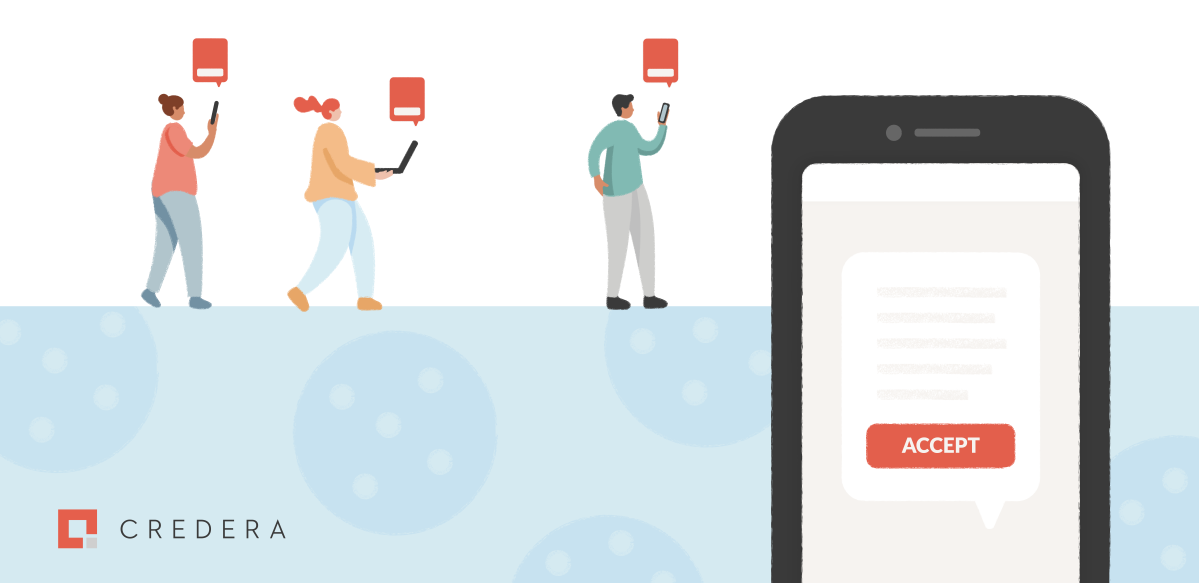
In part 1 and part 2 of this blog series, we examined what third-party cookies are, why they are changing, and some of the commonly proposed, but largely ineffective, alternatives the advertising industry is considering.
What Are High-Level, Future-Safe Data Strategies for This Change?
In this section, we will explore how companies that will make the most of the changing landscape are those that have a big-picture, customer-first mentality to forge strong connections and provide valuable experiences in exchange for their customers’ willingness to volunteer data.
Explicit Data Capture
Explicit data capture is a privacy-compliant and straightforward manner of determining more about a customer. Surveys and explicit preference choosing have been useful to marketers for decades. The main disadvantages of explicit data collection are that users may not know exactly what they are looking for, lie or skip through surveys, and will feel impeded in their tasks by direct requests for information.
Moving forward, balancing the pros and cons of explicit data collection will be vital to effectively create and maintain customer connections. One great way to start making this connection is a loyalty program (an explicit exchange of data for some incentive).
A successful industry example is Starbucks’ loyalty program. This leading coffee shop company exemplifies customer-centricity in their customer experience. This robust experience is credited with a significant portion of the company’s revenue growth over the last few years. By nudging the customer to engage with the loyalty program, Starbucks can gain additional information on user demographics and geographic distribution. This is used to track engagement behavior for ads served on their mobile app. All gleaned without the negative public perception of a company surreptitiously mining customer data or depending on third-party data.
Implicit Data Capture
Using varied paths to data collection helps ensure an advertiser can provide both what their customers say they want and what their behavior indicates they want, correcting for some of the blind spots in explicit data capture.
As an industry example, L’Oréal Paris uses both explicit and implicit data capture to create a more robust model for each of their customer’s needs. However, the differentiating factor in L’Oréal Paris’ data collection is in its in-app implicit data capture.
Creating a fun, unintrusive method of data collection allows for greater customer understanding and segmentation that is unaffected by third-party cookie deprecation or future legislation. Some examples of this are progressive profiling and in-app or in-site browsing patterns.
What explicit data capture lacks is the ability to anticipate what the customer wants before they know they want it. L’Oréal Paris’s solution to this problem was to acquire first-party data in more unconventional methods.
An augmented reality (AR) mobile app to virtually try on makeup is a great way to capture both ongoing preferences—where data from the makeup styles the users have tried is used to update their internal customer profile—and physical features—where data points captured on various physical attributes of users can be used to recommend products that others with similar features have tried and enjoyed. By leveraging in‑app browsing patterns and progressive profiling, L’Oréal Paris mixes diverse first-party data streams to reduce reliance on third-party data while maintaining a consistent customer experience.
Data Socialization
Another path to understanding customers is to uncover value in the data that is already in-house. Connecting siloed data from different streams will synergistically create value through customer behavior data enrichment to create a better customer experience.
For example, a customer data platform (CDP) is an industry-leading tool that enables the unification of existing data into a single location to inform business decisions and drive targeted communication to better serve customers’ needs. Combining existing data in new ways allows for greater customer segmentation within the user base and the extraction of more value without purchasing additional data enrichments.
Additionally, bringing more advertising data in-house allows for well-informed, data-driven decisions to be made without dependencies on digital technologies that may fall out of favor in the medium or long term, preventing the shift away from third-party cookies from happening again.
What Are Some Examples of Specific Ways to Implement These Ideas?
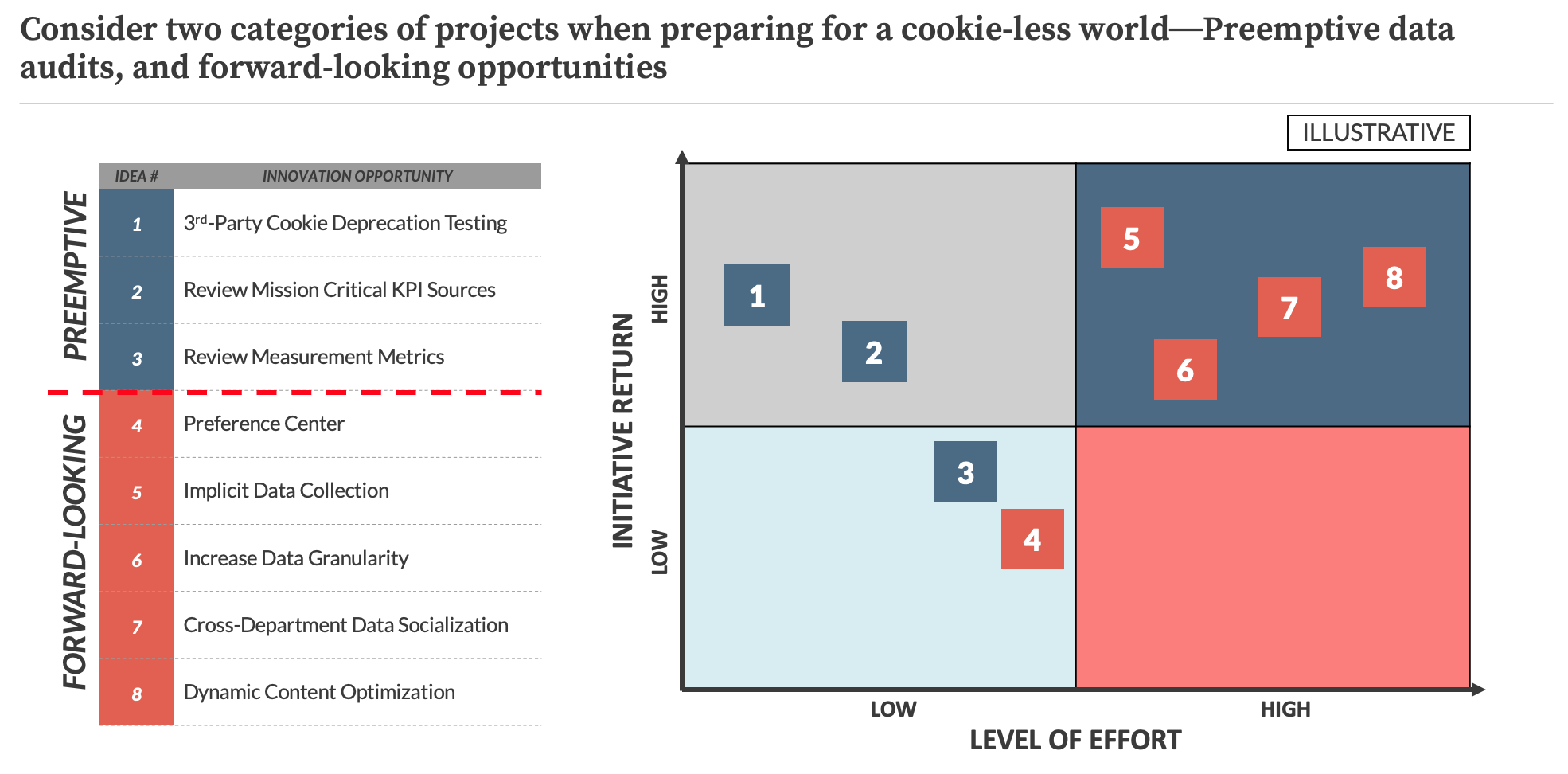
Cookie-less world preparations should be considered in two categories—preemptive data audits that measure and predict the effects of deprecation on different advertising channels, and forward-looking opportunities that increase competitive advantages.
Preemptive Data Audits
Ensure that dashboards, reports, and other decision-driving values will still accurately reflect real-world trends by performing preemptive data audits.
1. Third-Party Cookie Deprecation Effect Testing
Determining the extent of meaningful data collection that will change upon the deprecation of third-party cookies is an important consideration in creating a business response plan. Before deprecation occurs, this option would allow for empirical, business-specific data collection and comparisons to be run.
Additionally, further A/B testing could be done to determine the relative importance of specific data types from existing providers so that marketing budgets can be re-balanced depending on the structure of specific data ingested.
2. Review Mission Critical KPI Sources
Where do high-level guiding numbers come from? What are their sources and are there replacements for these data once cookies are removed?
Distinguish between KPIs and general metrics to focus energy into a sprint that will provide more information about the future of dashboards. Prevent dashboards from ‘flying blind’ using numbers that are not representative of valued metrics.
3. Review Measurement Metrics
This is a larger project scope than exercise two. Examine the dependence on third-party cookies to set a performance baseline. Grab dashboards currently in use and gather all metrics that are used for evaluation. Interview vendors in their responses to cookie deprecation and create a strategic plan to continue business operations without any lapses in business insights.
Forward-Looking MarTech and AdTech Opportunities
Maintain and increase competitive advantage by preemptively optimizing customer data collection, enrichment, and analysis.
4. Preference Center
Creating a fun, unintrusive method of data collection will allow for greater customer understanding and segmentation that is unaffected by cookie deprecation or future legislation.
One example of good preference center use is progressive profiling—asking for tiny bits of information at decision junctures. Incentive structures that include creative value exchanges such as early access to content or exclusive materials can be good additions to the classic discount incentive for encouraging users to provide more preference data.
5. Implicit Data Collection
Adding additional granularity in browsing and viewing pattern data collection allows for greater preference inferences. For example, gathering second-by-second viewer data can create internal profile preferences such as an aversion of sex scenes or preferences for colorful visual sets. This data, despite not being given directly by the user, can have as much or more predictive power on behavior than user-given data.
Unlike option four, preference center, creative incentive structures are not needed to nudge users to give data on conscious preferences—user behavior on its own is sufficient.
6. Increase Granularity of Data Collected
Encouraging users that share an account—shopping, streaming, etc.—to voluntarily separate their usage into profiles with attached information greatly increases the value in the data collected. With incentives such as more personalized recommendations or exclusive content, users can be encouraged to provide granular, personalized preference data without intrusive requirements or unwanted touchpoints.
7. Cross-Department Data Socialization
Using internally owned data across uncorrelated sources can create better customer profiles and understanding that will be unaffected by third-party cookie deprecation. If there is a possibility of socializing data across other business units, building out a procedure to increase data enrichment while maintaining customer data security could be a good option.
For example, sending hashed email addresses to be matched could maintain data security for both business units while increasing customer insights on both sides.
8. Dynamic Content Optimization
Using microsite advertisements to begin customer preference profiling from their very first interaction can increase the accuracy of customer lifetime value predictions.
For example, by creating multiple social media advertisements to target different potential markets and recording which advertisements users interacted with could allow for immediate recommendation profiling and customized landing pages without explicitly asking for customer preferences. These initial predictions can be updated as usual with further data capture for customer segmentation.
Taking Action
Could implementing a loyalty program, exploring more varied data acquisition paths, or unlocking more value from existing data be your business’ next steps? To learn more about the changing technology landscape, AdTech, MarTech, or any of the listed projects, reach out at marketing@credera.com.
Contact Us
Ready to achieve your vision? We're here to help.
We'd love to start a conversation. Fill out the form and we'll connect you with the right person.
Searching for a new career?
View job openings