Transformation
Sep 21, 2023
Unlocking creativity: Using structured innovation for data product development
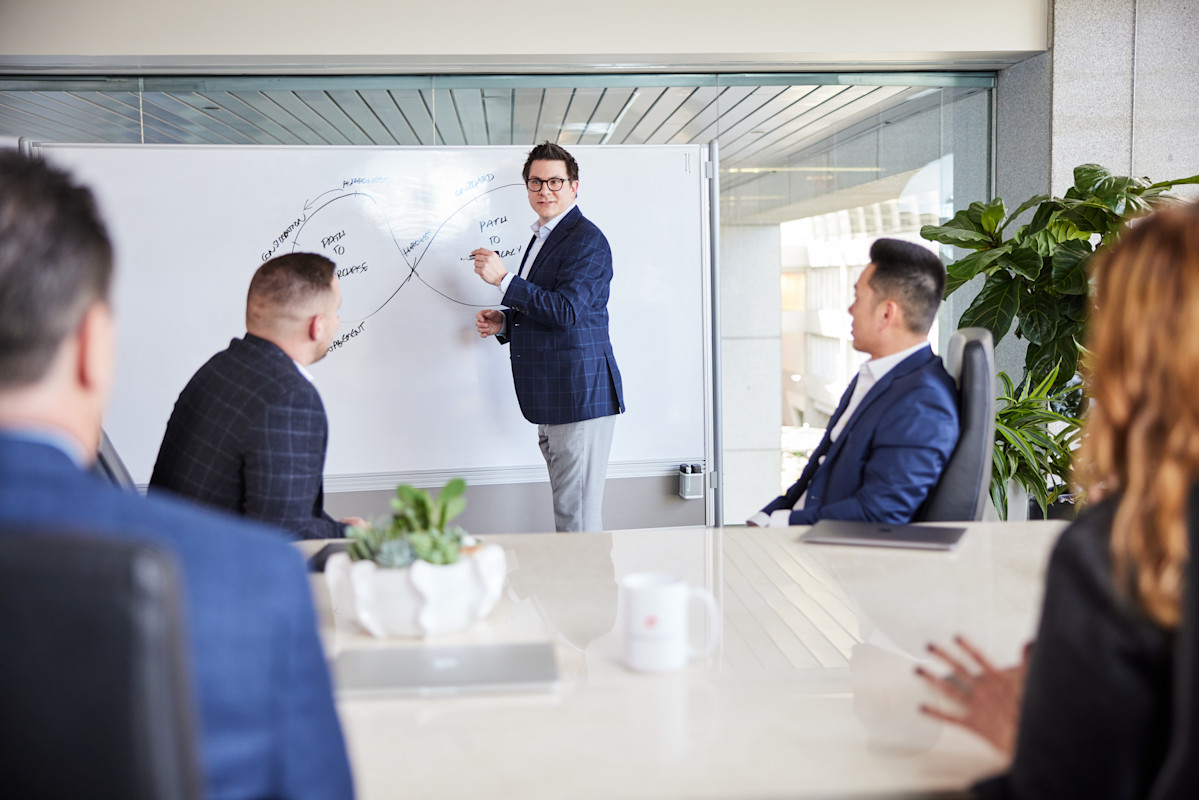
In today's ever-changing business world, the key to staying competitive can be found in unlocking data’s full potential. Developing new data products requires strategically aligning domain expertise with a structured approach, paving the way for innovation. By doing so, your organization can identify and capitalize on new opportunities to stay ahead of the curve.
In this blog post, we take a deep dive into the transformative potential of applying structured innovation to the development of data products. We will explore how the combination of design thinking and data science can generate novel solutions that lead to groundbreaking new products and help your organization shape its future.
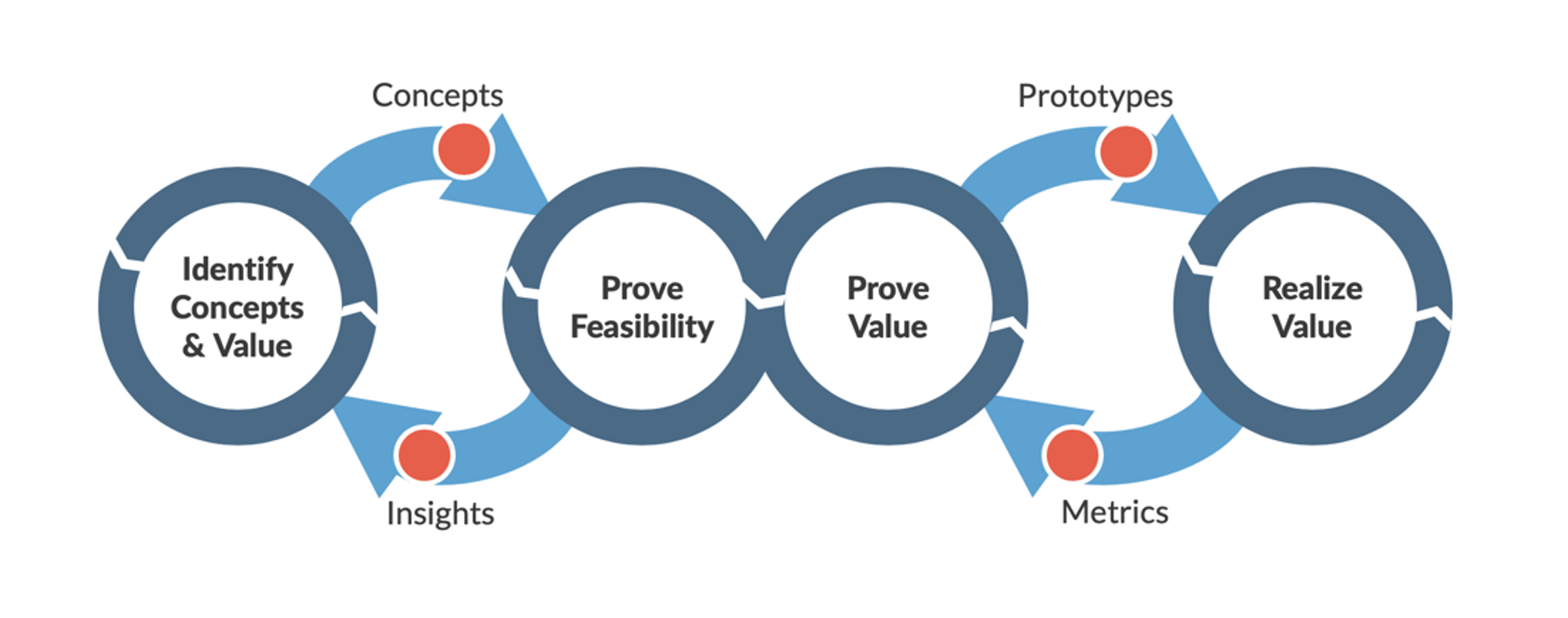
Figure 1: Using the data product development methodology to build a value realization pipeline.
Phase 1: Identify Concepts & Value
The first phase in creating a new data product is to identify your organization’s available opportunities. We recommend starting by hosting a workshop-style session with relevant stakeholders to generate options, casting a wide net before later narrowing down the pool of options. There are four key steps within this phase.
1. Generate
During this early stage, taking a strategic approach that looks at current pain points and envisions an ideal future state is key to generating useful data products. At Credera, we leverage several tools including theme occurrence matrices, sentiment distributions, and language analyses to identify opportunities.
2. Review
Once your team has generated an array of options, you may want to begin an objective review process based on a defined set of criteria, assigning each product a value under each category. The criteria may include potential business value, technical complexity, and feasibility risk. By performing this review, you will concretely understand which options are more fruitful and viable for the business.
3. Prioritize
After assessing each option in the Review step, we recommend prioritizing the various products according to the previously identified criteria. Once the list is sorted, stakeholders reach the key decision point of selecting the concepts they intend to attempt building. Sorting and then filtering down to a subset of high-impact products ensures resources are focused on the right efforts.
4. Prepare
In this last step, additional stakeholders are identified, necessary data is accessed, and platform and resource needs are finalized. You’re now ready to generate a proof-of-concept!
Phase 2: Prove feasibility
The next phase—producing a proof-of-concept through proving feasibility—is necessary in determining whether a data product is feasible and can deliver the value needed to justify the investment. Again, we take a similar structured process that begins with the end goal in mind: We recommend first defining, second developing, and third evaluating to ensure only the strongest ideas move forward through the innovation process.
1. Define
There are several cross-organizational steps that need to be taken when defining what a data product proof-of-concept should achieve. Initially, your team will work with relevant stakeholders to understand the high-level business objective, how the proof-of-concept will add value, and how it fits into the organization overall. Questions you will want to ask will range from technical infrastructure details to modeling strategies.
Consider the following:
What will the input and output data look like?
Where will the results be stored for easiest access and use?
What assumptions are we basing our thinking on?
Once you have your answers, then a data science hypothesis can be established for evaluation.
2. Develop
Before development can begin, a data understanding sprint is needed to identify trustworthy features that can be used to train models from the raw data. This sprint will assess data availability, data acquisition, data understanding, and data validation. Only when the data is accessible and understood is it possible to properly develop the proof-of-concept. At this point, your data scientists, data engineers, and designers can implement the product, typically a machine learning model or dashboard, and perform any user testing.
3. Evaluate
Assessing the data product is the end goal of the proof-of-concept phase. This is when the business stakeholders from the Define step evaluate whether the product fits their needs and delivers the value they were looking for. This is another critical decision point in the structured innovation process where the team must decide whether to continue iterating on the product (persevere), make fundamental changes (pivot), or bench the product completely (pause) before moving on to the next phase.
Phase 3: Prove value
Following your team’s decision to persevere, pivot, or pause, we enter the next to last phase: proving value. At this point, you should have a working product ready and should begin contemplating how said product would integrate with existing parts of the business to drive key metrics. Again, we follow a series of four steps.
1. Prototype
Knowing that the product in question is feasible, your team should begin executing product sprints with the aim of rapidly prototyping and testing to drive test results in as little as three to five days. Using an ideate/design/low-fidelity/high-fidelity approach, the goal is to quickly validate the potential product with stakeholders and adjust accordingly, thus achieving maximum results with minimum effort. This saves both time and resources, allowing for more productive throughput.
2. Validate
With the test, or “high-fidelity” prototype, the sprint team further validates by proactively considering change management efforts, capturing user feedback, and validating business hypotheses. Here, barriers to change and potential shortcomings are identified, and business uses are compared to those originally intended. These inputs allow the team to extrapolate.
3. Extrapolate
The extrapolation phase finally documents additional use cases from user tests, evaluates risks (both ethical and compliant), influences experiment design, and adjusts the business case once more. Here, the team once again reaches a persevere, pivot, or pause decision point—either returning the minimum viable product (MVP) back to prototyping (pivot), advancing to the production phase (persevere), or ceasing experimentation (pause).
4. Productionize
Should the experiment reach the production phase, the team will then componentize, performance tune, create production architecture, and generate an MVP plan, leading to the final “go/no-go” decision.
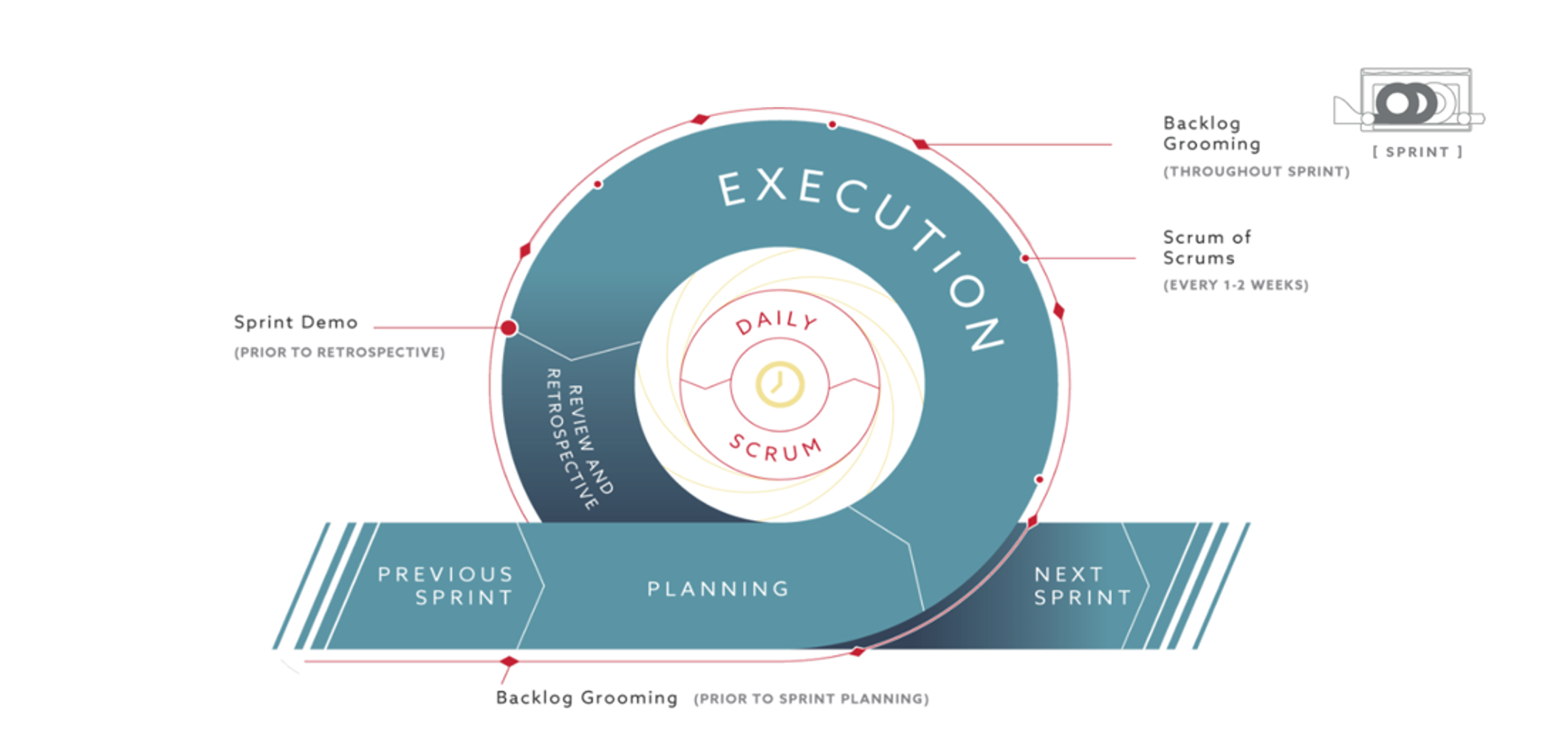
Figure 2: Implementing sprint cycles in the agile development implementation phase to expedite value realization.
Phase 4: Realize value
Now that the product has proved valuable for the company, a continuous experimentation approach is employed to take the data product to production. This is done by breaking the work into sprint cycles following a standard plan/execute/review/revise format, thus allowing your team to deliver solutions quickly, respond to changing requirements, and realize value with more confidence. The team measures impact with each stage, ultimately leading to the final decision to execute a full release.
The team can use a “build, commit, and measure” process to progress to realizing value.
1. Build
During this build phase, we can further divide the approach into iterative sub-phases: plan, execute, and review and revise.
A. Plan
Using the proof-of-concept, it is now possible to identify functionality that is ready for release. Components that are under development are planned and responsibilities are distributed among the coordinating teams. This will be translated into a backlog of work items that is managed by a product owner who is ultimately responsible for delivering said product. The feature development stories are then ordered by priority and set as sprint goals.
B. Execute
To facilitate the development of the prioritized features, the team holds daily meetings called “scrums” to cover progress, future plans, and any blockers stalling the team’s progress. Additionally, the development team will implement and test the new functionality, ensure the product is behaving as expected, and reprioritize the backlog as needed.
C. Review and revise
Once a specific feature has been completed, the team reviews the product’s functionality with the product owner, usually culminating in a demonstration for all internal project stakeholders. There, feedback is collected and analyzed to determine if further changes are warranted. If changes are identified, the team writes new work items to be conducted in a subsequent sprint; if the team does not call for further revisions, however, you are ready to move to the release cycle.
2. Commit
At last, the long development process is finished, and it is time to release the product. The last sprint—the “release sprint”—validates all new functionality, gains stakeholder approval, plans new feature support, and finally pushes new functionality to the proper environments. Additional support activities may include assembling proper documentation for internal knowledge transfer and writing release notes for users to understand the new changes.
3. Measure
Finally, software is released into a suitable testing environment where it is run until a statistically valid sample is gathered. Results are analyzed, and if the desired results are achieved, you have the green light: Promote the product up to production environments and deploy new functionality to the end users. Congratulations!
Moving forward with innovative data product development
In this blog post, we’ve explored how utilizing a structured innovation approach can help create value more quickly while reducing unnecessary spending and resourcing. By generating ideas tied to business outcomes, testing both feasibility and value, and executing product components in an iterative manner, your organization will be well-equipped to create valuable data products.
To learn more about building data products, we invite you to explore Credera’s data science service offering or to reach out to us at marketing@credera.com.
Contact Us
Ready to achieve your vision? We're here to help.
We'd love to start a conversation. Fill out the form and we'll connect you with the right person.
Searching for a new career?
View job openings