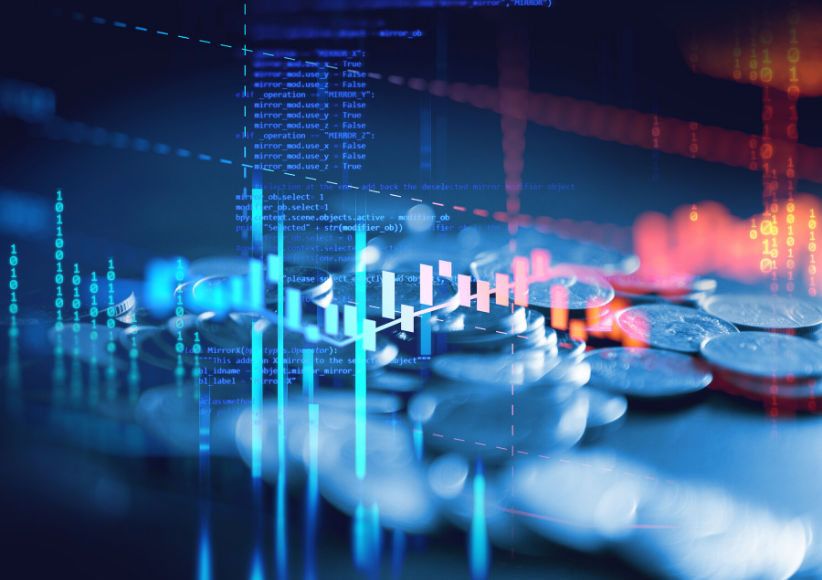
Recent trends in the financial industry show companies value integrating everyday business activities with machine learning. Machine learning is a subset of artificial intelligence that creates models that learn and predict outcomes with little to no manual coding. Gartner estimates that globally there is $2.9 trillion in business value to be gained for businesses that augment with machine learning by 2021. Furthermore, 75% of executives feel they risk going out of business within the next five years if they don’t integrate with machine learning. Yet, despite recognizing the importance of these technologies, many companies struggle to implement them, with 80% of companies failing to even have their machine learning project get past the proof of concept stage.
We’ve worked with clients across a range of industries by implementing scalable solutions and equipping them with the tools for lasting success. In the financial industry, we have found success integrating machine learning in many use cases, but the following are great places to start:
Fraud Detection
Data Entry
Document Classification
Customer Personalization
Underwriting
Customer Service
The following sections of this blog will highlight how machine learning is used in each of these use cases, and the business value that can be gained.
1. Fraud Detection
Real-time detection of fraud is a significant way for financial institutions to reduce costs. The Association of Certified Fraud Examiners (AFCE) found in 2016 that the yearly median cost of fraud within a business was $150,000, where 23% of these organizations experienced losses exceeding $1 million. Similarly, the FTC reported in 2019 that 1.4 million customers were the victim of fraud in the prior year, totaling $1.5 billion in costs. However, not only is it important to detect fraud, but it is critical to correctly diagnose fraud – MasterCard reported in 2016 that 1 in 3 customers that were falsely identified for fraud did not return for future business.
Traditionally, fraud detection is handled through rule-based systems that can require extensive logic and can be difficult to personalize by customer. However, through machine learning, fraud detection can be personalized on a customer-by-customer basis and allows traditional business rule-based systems to transition into a support role. Machine learning fraud detection systems ultimately offer higher performance than their rule-based counterparts as seen in this study by Capgemini in 2018 where machine learning detection systems were found to correctly identify fraud 90+% of the time, while also minimizing the time to investigate by 70%.
These systems utilize a constant cycle of monitoring, detecting, and learning to build predictions. This allows fraud detection system to be real-time, and always current. These systems learn and predict using various machine learning models, where the common ones for fraud detection are random forests, support vector machines, k-nearest neighbors, and neural networks. A great example of a company using a machine learning based system is PayPal, who utilize an ensemble of a linear machine learning model, neural network, and a deep neural network to separate suspicious transactions from safe transactions. These suspicious transactions are then sent to a different workflow to go through additional verification steps.
2. Data Entry
We highlight in our Data is the New Oil series, data quality is one of the four biggest issues preventing organizations realizing analytical benefits, with Gartner reporting in 2018 that organizations believe they lose $15 million per year due to poor data capture. People can be prone to error when doing repetitive and tedious tasks such as data entry. However, improper data entry will reduce data quality and result in poor decision-making as bad data will yield bad predictions. Poor data quality can also reduce productivity as workers will spend more time cleaning and validating the data, and hinder marketing outcomes as audiences can’t be properly targeted when using bad data.
Machine learning can be used to improve this process by automating portions of the data entry workflow to ensure the critical details are captured. This can also increase the data entry accuracy while simultaneously making the whole process quicker. A common example of machine learning being used for data entry is the processing of checks. Since checks contain a static layout, computer vision technology within machine learning can easily read a check to detect the amount, account number, etc., and immediately begin processing without human intervention.
3. Document Classification
Similar to data entry, document classification is another area where machine learning can improve overall quality while simultaneously reducing costs. Financial institutions can remove the human bottle neck of processing and labeling text documents by automating the process through machine learning. This process can significantly reduce the processing time for labeling documents. For example, the technology firm AxisAI reported in 2019 they were able to classify over seven million unstructured documents in 28 days by utilizing machine learning.
Document classification works through a two-step process, where first the document is read using a technique called Optical Character Recognition (OCR) that digitizes the text on the document. The output of this process then feeds into another machine learning model that reads the text to determine the context and applies a label to the document that is relevant to the business.
This process can also help reduce physical storage space of documents. Since OCR digitizes the text, the documents can then be stored within the cloud, thus making it readily available to employees across the company, and also makes the documents easier to search.
4. Customer Personalization
Financial institutions are being surpassed by retailers and tech-savvy companies that put personalization at the core of their business models. Recent research shows that 1 in 5 people are open to changing from their existing bank to a big tech company such as Amazon or Google due to the personalized experience, and that two-thirds of Amazon Prime customers would bank with Amazon if they had the opportunity. Improved personalization allows financial institutions to better focus their marketing efforts and next-best offers. It allows organizations to predict customer interactions and anticipate individual needs, building a deeper relationship with the customer. In short, enhanced personalization can boost sales, improve overall customer service, and trigger targeted financial advice in real-time that McKinsey estimates can yield a 5 to 15 percent lift to revenues.
These tools work by understanding a customer’s past and recent activity to build a picture of the customer. This picture can then be augmented by including weather, location, customer’s risk aversion, current stock market, and other external factors to give strategic recommendations. For instance, if a customer uses their credit card or bank card to book a flight, the machine learning model could suggest a relevant and personalized hotel or car service offer that would be linked to the respective card.
Personalization also helps combat churn by helping to identify customers that are at a risk of leaving through personalized churn analysis. This process leverages historical customer activity to build a model of in-depth personas that will help your organization understand why and at what point in their customer journey a customer left. This model can then be used to identify potential customer churn due to upcoming fees or reduced activity and possibly initiate a retention-based call with a local branch.
5. Underwriting
The core of an underwriter’s job is to accurately gauge risk on a per client basis. This work can involve significant amounts of exploratory analysis against large datasets of various file formats to ultimately price the risk. This can be tedious, yet machine learning allows this process to be automated and simplified. Models can determine high-risk customers and immediately reject them, while also identifying low-risk customers for approval. It also allows policy pricing to be tuned to each individual client, similar to the previous personalization section. These improvements are expected to bring significant cost savings in the coming years. For instance, Juniper Research expects savings will reach $2.3 billion by 2024, up from $340 million in 2019.
Machine learning shifts the scoring process in underwriting away from traditional models, such as the FICO score that is considered “okay” for everyone but “excellent” for nobody, to more personalized models. These personalized models can surface hidden features during the scoring process that help detect minute differences between borrowers that historically would have been considered the same. This helps boost overall revenue as institutions can now customize interest rates and approve borrowers that were previously considered “credit invisible.”
6. Customer Service
Back in 2018, Gartner found that organizations that implemented machine learning in their customer service workflow saw a 70% reduction in call, chat, and email inquiries. A common example of machine learning improving customer service is through chatbots. Chatbots are relatively inexpensive to implement and allow customer service to be available 24/7/365, which can be a difficult feat to achieve for human-led teams. Chatbots have also been found to reduce customer interaction time and boost overall customer service, which is crucial to overall revenue according to a study by Zendesk. Zendesk found that 42% of customers were more likely to return after experiencing good service, while 52% chose not to complete their purchase after a single instance of poor service.
Chatbots can be either voice-based or text-based, where the former involves a customer interacting with the chatbot over the phone and the latter has the customer interact through the business’s website. These services allow commonly asked questions to be instantly answered as well as pre-screen customer issues so agents can be more targeted on their calls. This also helps free up agents to focus on more complex tasks and high-margin customers.
Outside of chatbots, machine learning can be used to analyze caller information to find their purchase history, whether they are a high spender, and other characteristics to match them with an agent with the best set of skills to assist. It can also be used to help counteract churn by analyzing recorded calls for changes in voice inflection to determine when and why a customer became frustrated.
Going Forward
Machine learning is a fascinating technology that can provide many benefits for any organization. We at Credera would love to help your organization understand how you can leverage machine learning to achieve these benefits. Please reach out to us at marketing@credera.com to start a conversation.
Contact Us
Ready to achieve your vision? We're here to help.
We'd love to start a conversation. Fill out the form and we'll connect you with the right person.
Searching for a new career?
View job openings