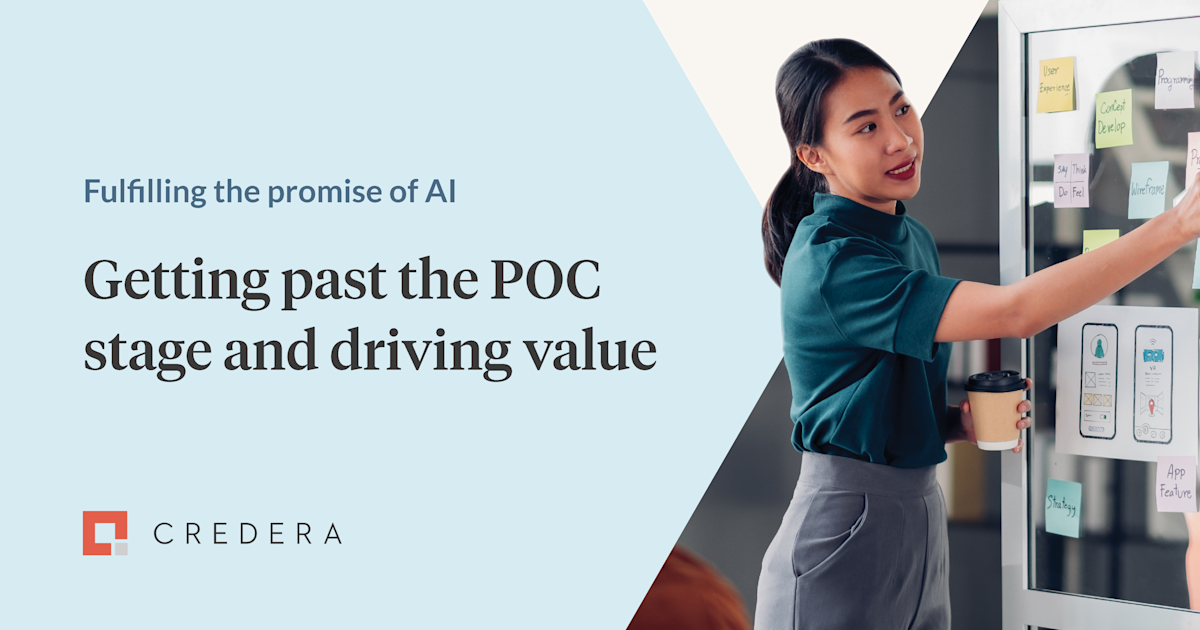
It’s a harsh reality of the initial stages of the AI era: A significant amount of money and hard work will go into the creation of a large number of AI-based initiatives, but the vast majority will never make it past the proof-of-concept (POC) stage.
Peter Bendor-Samuel, CEO of Everest Group, wrote in Forbes that up to 90% of generative AI POC pilots created by large organizations won’t move into production any time soon, and some may never get there.
Here we’ll explore some of the reasons all types of AI-based projects linger in no-man’s-land, along with a few suggestions for keeping your organization out of the same traps.
The hurdles
The key to launching successful POCs is figuring out which ones will likely drive the most value and investing solely in those to get them into production. But one primary issue with many POCs is that they were never designed with the idea of being in production and driving value to begin with—technological or economic constraints of the real world prevent them from being valid enterprise-grade solutions. That lack of foresight may be unintentional, but it also could be due in part to the “innovator’s dilemma.”
The innovator’s dilemma—the title of an influential book by Harvard Business School professor Clayton Christenson—is the idea that while large organizations have some motivation to innovate, they’re more concerned that any new products will undermine their existing ones. There’s not much incentive for those new solutions to succeed.
Another major obstacle for large corporations is their traditional methods of operation. A typical project might begin with a six-month research phase to gather the right requirements, followed by a three-month vendor evaluation. Then, after a six-month implementation, who knows what the AI landscape looks like? The project is out of date before the teams have completed the first month of that year-plus project.
The solution
For most organizations, success in this space requires a significant shift from the operating model described above to a model of rapid prototyping, which is doing a whole series of small, real projects quickly as experiments, learning from them, and throwing most of them away. The common thread among companies that launch successful, value-driven POCs is that they maximize optionality as they begin the process, by building small, cheap, scrappy POCs, then testing and learning as much as possible from them.
The thing is, you can't think your way into acting differently—you have to act your way into thinking differently. To do that, you’ll need to stretch and build different organizational muscles, which means rethinking and reinventing entire processes, from the way projects and roadmaps are planned and funded to how teams are structured to how decisions are made.
Of course, as part of the natural evolution of this type of change, some area of your new system will ultimately break down and become the new constraint, the new bottleneck, so you’ll need to quickly identify the new blockers and solve for them. This is yet another reason organizations should begin to ingrain AI into their operations sooner rather than later.
(For a deeper dive into how to position your organization for success, check out our article “Who owns your organization’s AI strategy?”)
Characteristics of successful AI initiatives
Successful AI projects have the following qualities in common:
Performance: Your AI initiative has to move the needle in a measurable way, such as handling repetitive tasks to increase productivity, detecting anomalies and reconciling discrepancies to keep your data clean, improving customer service with chatbots to free up representatives for other tasks, or enhancing customer experience with targeted messaging that boosts conversions.
Security: Your technology, as well as your processes and workflows, should be designed to make sure the integrity, availability, and confidentiality of your AI initiatives aren’t compromised in any way. Special consideration should be paid to keeping your data safe. This means taking steps to prevent leaks of proprietary information through answered prompts, secure the data used in training AI models, and implement defense mechanisms against malware, among other precautions.
Scalability: An AI-based endeavor that works well in one department or business unit should be easily adapted for deployment in others. If its capabilities are limited or too specific to one area, your strategy and planning went awry somewhere down the line. A good way to ensure that your AI projects can scale and evolve is to build them in a modular way so that as technology advances and changes, they can be revised to leverage that technology.
The bottom line
If your organization is having a tough time bringing AI initiatives to life, especially in a meaningful way, you’re not alone. New frontiers require new mindsets and new approaches, and that’s a difficult ask for a lot of companies.
A knowledgeable, experienced partner can help. While many consultancies provide generic, high-level solutions, Credera develops specific, actionable plans our clients can follow, with realistic, detailed solutions to their AI-related issues. Schedule a call with our specialists to get started.
Contact Us
Ready to achieve your vision? We're here to help.
We'd love to start a conversation. Fill out the form and we'll connect you with the right person.
Searching for a new career?
View job openings