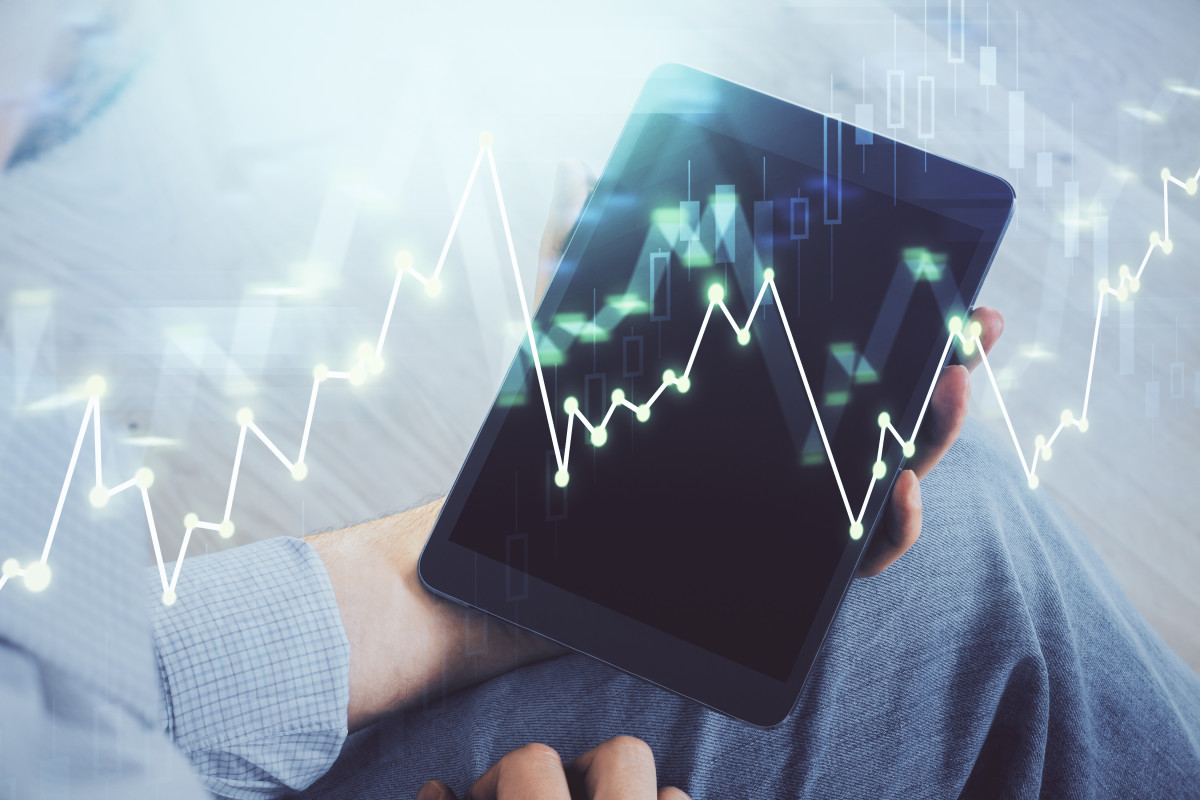
Sales is considered one of the oldest professions in history. It is constantly changing and evolving, utilizing more and more modern technologies, including the integration of machine learning (ML), a subset of the artificial intelligence (AI) universe of applications. Countless reports and statistics have shown that the use of AI brings huge benefits for sales organizations:
An increase in leads and appointments of more than 50%, cost reductions of 40% to 60%, and call time reductions of 60% to 70%.
High-performing sales teams (top 24% of more than 2,900 sales professionals surveyed) are 1.5 times more likely to forecast using data-driven insights.
30% of business-to-business companies will employ AI to their sales processes by 2020.
At Credera, we are experienced with providing data-driven solutions for sales teams across many industries. Since it might sound abstract to talk about the concept of machine learning in sales, let’s dive deep into a day in the life of a salesperson and look at where machine learning could fit in:
1. Automation
A typical day of a salesperson usually starts early and may continue into the weekend. 76% of salespeople rise before 7 a.m., 35% even wake before 6 a.m., and checking their email is the number one priority. In addition to the early hours during the week, 61% of salespeople work weekends. Sales is a stressful job with high workloads, some of which can be highly repetitive and mundane. Salespeople spend 33% of their day writing emails and scheduling calls, 17% entering data, 12% on internal meetings, and only 17% prospecting and researching leads.
Machine learning can help automate highly repetitive workloads. For instance, machine learning allows for the automation of welcome emails to new leads, demo emails to new sign-ups, and follow-up emails to non-responsive emails through a process called natural language processing (NLP). This process commonly handles text-based querying to provide real-time responses but can also be utilized to understand spoken words through voice recognition applications. Great examples of voice recognition being utilized with automation are Amazon's Alexa and Apple's Siri, which enable automated scheduling, replying, and data entry.
Machine learning can help reduce a salesperson's time spent on mundane tasks by 50% and allow them to focus on tasks such as providing a better experience to existing customers or building relationships with prospects.
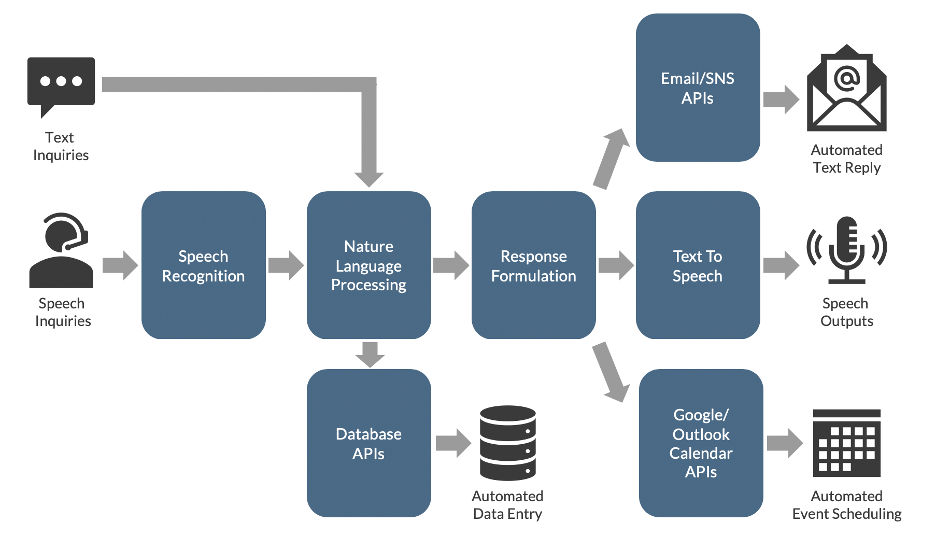
Illustration of ML-based automation in sales
2. Filtering Insights
After replying to emails early in the morning, a salesperson usually starts to research new leads. Among all the ways of prospecting, social media ranks first in time spent. Social media usually contains an enormous amount of data, which could be both a good thing and a bad thing. On the bright side, there are many potential sales opportunities. On the other hand, there is so much unrelated information, it’s difficult and time-consuming to filter useful insights.
Modern-day AI and ML technologies excel at recognizing patterns. Social media data usually consists of images, texts, and other features. Using computer vision (a process where a computer processes and analyzes images or videos) and natural language processing modules, we can leverage this data to capture the slightest patterns and insights that can help drive new business. With a continuously updated dataset, we can easily filter unrelated information and help identify what could lead to new prospects.
For example, Q-Platform—an AI and analytics tool built by our sister agency, Sparks & Honey—uses big data and human intelligence to quantify and predict cultural changes on different social media channels. It uses a robust data pipeline of cultural signals (that includes news articles, academic discoveries, patents, venture capital activity, social media posts, and more) and contextualizes it through artificial intelligence. Q-platform reduces months of laborious social media researching into just hours of analysis while also improving the accuracy and consistency of identifying leads.
3. Optimization
If you chat up an experienced salesperson any time throughout the day, you’re likely to hear a list of dos and don’ts that help with sales. Some of them could be useful, while others could be based on subjective experience or even superstition. In a recent Salesforce survey, 25% of salespeople said they’re superstitious about selling and 66% of them find magic in the coffee they drink.
An ML-driven sales practice principal based on historical customer data and predictive analysis modeling could be really useful in this case. There are certain actions you should avoid, such as calling the customer every five minutes until they buy, and certain actions you should target according to the type of customer. Knowing the correlation between actions and customer behaviors, a sales manager can organize the team better, bring higher close rates, and increase performance. This correlation can also be leveraged to drive personalized approaches for sales on a customer-by-customer basis. For example, Company A expects a daily phone call to discuss a new topic while Company B would like a weekly email.
Throughout any typical day, salespeople are entering data, creating reports, and sharing results in meetings. In addition to optimizing the actual sales practices, machine learning can apply to internal tools used by salespeople. Adopting a cloud software service, also known as software as a service, can help to optimize the internal reporting process, reducing the time spent on unnecessary internal meetings. Visualization pipelines can be set up with updated data for generating everyday sales reports. More importantly, forecasting can also be done in a more accurate and efficient way, as explained below in the forecasting section later in this article.
4. Pricing Strategy
A good sense for pricing is a must for any salesperson. At some point, they’ll have spent time pricing the products they’re selling. Traditionally, the sales and marketing team found successful pricing through trial and error. Before the prevalence of the internet, markets used to be relatively isolated and pricing was manageable with local trials. However, given how easy it is for a customer to compare prices and learn about trends, it is difficult to predict future demands based on trials alone.
Machine learning can be of great help here. In most cases, machine learning finds the sweet spot on the demand-supply curve, quantifying the price elasticity of demand and maximizing the revenue. A lot of salespeople are already using such tools: a trade-in manager at a car dealership gathers information from the mechanic and feeds it into a machine learning app to get your trade-in price. The pricing of an upcoming product considers factors such as competition, season, special events, operating costs, and macroeconomic situations. With the help of machine learning, the right pricing ensures products or services sell fast and are profitable at the same time.
5. Forecasting
After a busy day checking emails, making phone calls, and researching new leads, a salesperson may have to attend internal meetings before completing the workday. One of the tasks here is internal reporting and forecasting. This is where the sales manager weighs in all the factors, listens to fellow salespeople’s opinions, and makes forecasts on the demand for products and services. Traditionally, they rely heavily on spreadsheets with disconnected data, where the process is highly inaccurate and manual. With the introduction of a customer relationship management (CRM) technology, a centralized repository is provided that can be utilized for machine learning.
Demand forecasting is one of the major objectives for a CRM. If integrated into a CRM with updated and automated pipelines, sales forecasts can be automated and optimized for salespeople without much effort. The data-driven forecast can be used as a benchmark, as the usual level of business, and help with setting short-term goals. It can also be used for planning budgets and strategies. With a more accurate forecast, the sales team could increase its profit and strike the balance between demand and supply.
Churn and customer lifetime value analysis are also available through the use of machine learning with sales activity data and sales engagement data. This allows companies to know how much revenue they can expect from a customer throughout the business relationship. When using supervised machine learning models with labeled historical datasets on customer churn, the sales team can focus more on customer satisfaction and customer retention. Customer satisfaction is now the primary measure of sales success, followed by team and individual quotas. Using advanced forecasting models, sales teams can aim for higher customer satisfaction and an overall increase in sales performance.
Next Steps
Going through a general day of a salesperson, we can see how much machine learning helps to save time and boost productivity. Utilizing data gathered from sales activities, machine learning techniques can bring revolutionary changes to sales performance in almost any industry. If you are motivated to implement this change or have any questions regarding machine learning in sales, please reach out to us at marketing@credera.com.
Contact Us
Ready to achieve your vision? We're here to help.
We'd love to start a conversation. Fill out the form and we'll connect you with the right person.
Searching for a new career?
View job openings