Data
Apr 20, 2020
Data Integrity Series: Three Key Factors to Incorporate Into Your Data Quality Approach
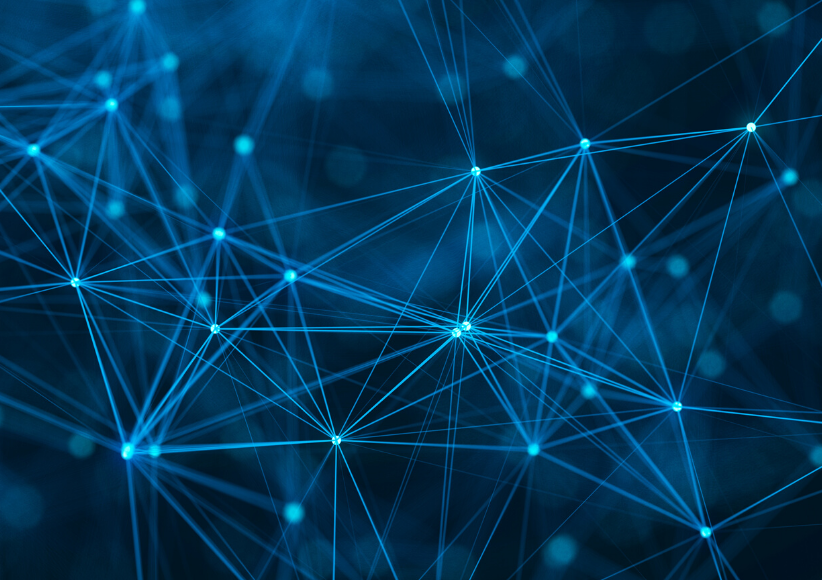
How are we still talking about data quality? For 15 years, every client I’ve engaged with has been limited in some way by the quality of their data. Issues arise from poorly architected applications, insufficiently designed data pipelines, or a lack of data governance and standards across their organization. And these problems have worsened over time. Organizations are still facing a battle against data quality, but they are doing so with 100 times the data being collected from 10 times the number of sources.
To further complicate matters, a standard approach to data quality isn’t working anymore. In my past life of enterprise resource planning (ERP) implementations, we used to evaluate quality, field by field, mapping values to make sure that data from legacy applications was clean to push into SAP or Oracle. We had weeks or months to manually remediate the data and build automated steps to clean the data through transformations. Today, data science and machine learning models fail to accurately predict outcomes that drive real-time decisions because of poor quality across a combination of features throughout multiple first- and third-party data sources. When any one of many attributes are missing or contain corrupt data, our models can be either poorly trained or our inference is thrown off.
When we think about data quality, the traditional data quality elements of accuracy, completeness, integrity, timeliness, uniqueness, and validity are where we should all start (as discussed in the first blog post of this data integrity series). But there are three additional factors that we should understand when building a foundation for our data quality processes. These factors are data lineage, data origin, and data use.
Data Lineage
Much of an organization’s data jumps through various hoops and transformations to get to its final resting spot. Like software development, when evaluating quality, we must identify failure points in the data flow upstream of the target data store. For many organizations, asking for a mapping of data lineage for their enterprise data is like asking my 8-year-old for directions to California. They can point to the location on the map, but they can’t give turn-by-turn directions.
Good news for the directionally challenged—tools designed with AI and crowd-sourcing capabilities are available to map data lineage similar to how Google Maps can tell my daughter how to get to California. With tools like IBM Knowledge Catalog, Collibra, and Data.World, business users can visualize the interdependencies of the data in a data catalog. Data catalogs work to organize data sets in your organization and enable searching for specific data so users can understand its data lineage, sources, uses, and the data’s value. Data lineage visuals provide a roadmap of data consistency, accuracy and completeness, which enables the user to better understand and trust their data. For the end user to trust their data, they must know where their data comes from, where it’s been, how it’s being used, and who is using it. Data lineage is the key.
Data Origin
One of the key roles of data lineage is the identification of your data sources to determine the origin from which your data is created. Understanding the role of your source systems in the creation of data is key to data quality. Data from internal applications will need to be governed differently than the way third-party enrichment sources are governed. Data originating from critical transactional systems that are subject to audits need more controls and higher data quality than operational data systems.
Tell me if this sounds familiar: Your IT department begins a data centralization project by documenting all your source systems. They proceed to document a list of attributes, mapping common attributes into a massive Excel spreadsheet, and creating source-to-target mappings that will be handed off to a data engineering or business intelligence team to develop ETLs and/or data pipelines to move data into data lakes, data warehouses, data marts, and visualizations. After a year (or longer) your team has deployed and distributed dashboards, visualizations, and reports throughout your organization and ties a nice bow around the documentation and stores it on SharePoint, Confluence, or a shared drive. Now, fast forward six months. Your team has been redistributed to other projects, you’ve restructured your SharePoint libraries, and marketing has added five new sources of customer data they want centralized and included in their single view of the customer to analyze. Tickets are created with new requirements, a new team is formed, and a discovery exercise commences to identify the sources, documenting attributes and mapping common fields, and building new source to target mappings. The cycle continues.
Understanding the point where data in your analytics layer originates is key to ensuring data quality. Rather than cleaning up the data once the mess has been made in your data warehouse, data quality should begin at the source. Having a data catalog and the tools to identify the point of origin of a field simplifies the processes necessary to make future changes to your analytics engine when the business requires a change. Furthermore, a data catalog that is business facing decreases the burden on IT to find and answer questions from users about how KPIs and metrics are defined and how they should be reconciled to the source systems they have access to.
Data Use
The KPIs and metrics used by the business and the models built on analytical data platforms should drive your data governance and data quality priorities. Knowing how data is going to be used allows the data stewards to focus on monitoring quality and cleansing the right fields so time isn’t wasted on building rules and monitoring unnecessary data transformations and fields. For example, your marketing organization may be collecting hundreds of data points about your customers. If 15 of those customer attributes are passed to trained machine learning models for determining segmentation and “next best action,” it is likely more important to ensure high fill rates and high quality for those specific fields than the others.
Connected teams of business and IT representatives can prioritize critical data in your systems and ensure the focus is directed appropriately. The data catalog should highlight these critical fields, transformations, and source systems and applications to keep your priorities on the right data. So often, time is consumed with QA and remediation on data that doesn’t drive decision making and outcomes. Having a focus on the right usage of data is critical to a successful data governance program.
Your Data Quality Process
Don’t take any more time away from your organization with a poor data quality process. Using tools and processes to manage your data governance efforts by focusing on data lineage, data origin, and data usage, in addition to the traditional data quality metrics, can reduce costs and free up time for increased value added to your organization. The approach of Credera’s Data & Analytics Practice to data quality and governance can help identify where we can optimize and improve these processes. If you’re interested in learning more, please reach out to us at marketing@credera.com.
Contact Us
Ready to achieve your vision? We're here to help.
We'd love to start a conversation. Fill out the form and we'll connect you with the right person.
Searching for a new career?
View job openings