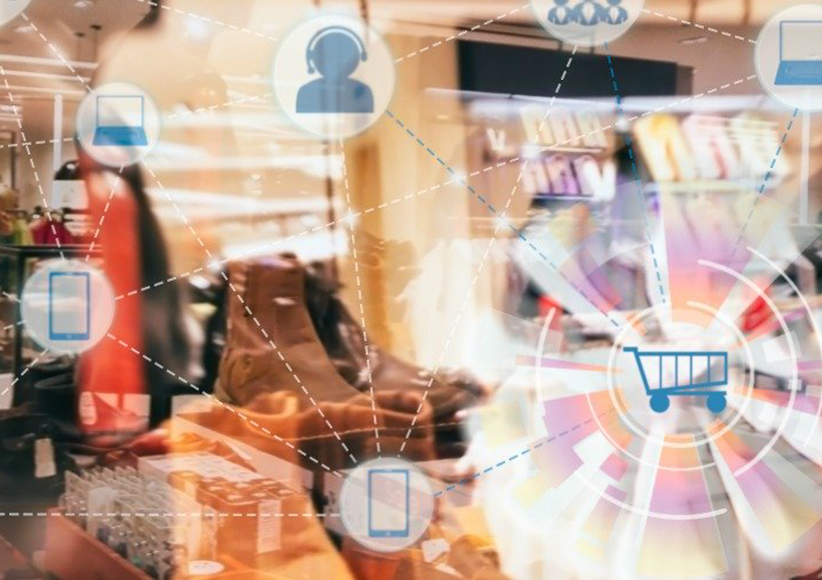
Brand marketers must deal with an ever-increasing amount of data. The expanding data cache includes mobile, search, social engagement, brand awareness, campaign effectiveness, browse behavior, and website conversion. This data is often sliced and diced across campaigns, channels, product categories, and geographies. Ask any marketer about the ROI for their latest campaign and you’ll likely get a very precise answer.
The same does not always hold true for customer analytics. Sure, marketers have purchase and browse data for most customers. And they often have email engagement data and loyalty program data for a significant percentage of customers. This customer data is invaluable for segmentation, re-targeting, and list optimization purposes. But ask a marketer for customer metrics like the average customer lifetime value (CLV) for a recent acquisition campaign or the millennial response rate to a new product launch, and you’ll likely get a stumped look.
Brands have realized they are competing on customer experience, not just product and price. So they are investing more in customer data and applied analytics. The successful few in this realm (Amazon, Netflix, Sephora) are taking market share from the rest of the pack. Many legacy retailers are struggling with silo-oriented processes and organization structures that impede the full potential of their customer data. According to a recent McKinsey study, retailers are realizing less than 40% of the potential of customer big data for driving revenue and margin growth.
Key Challenges
Measuring these qualitative customer metrics is not always a simple proposition. For many brands, customer insights are spread across product or marketing channel silos. This makes it harder to build and apply cross-channel customer analytics. A good place to start is gaining alignment on who has accountability for full customer lifecycle metrics.
Another challenge is creating a single 360-degree view of the customer. This involves moving beyond purchase data to incorporating data across the customer journey. This journey data includes mobile app, product reviews, social engagement, customer care, and various other types of third-party data. Much of this “big data” does not easily integrate into a traditional data warehouse. The data can be unstructured, sent via API, real-time (vs. batch), and have varying degrees of sparsity. Customer identity management is critical here. Emerging toolsets called customer data platforms (CDPs) can ingest a wide variety of customer data sources, then “stitch” the various data points to a single customer ID, and finally, prepare the data for downstream marketing use cases.
Once the 360-degree customer insights are integrated into a single view, the data needs to be integrated with customer-facing marketing systems. This gets complex quickly. Fortunately, automated business rules can be applied that predictively “score” and determine the next best customer actions (content, offers) across digital channels. Emerging machine learning/artificial intelligence software can be applied to automate the predictive scoring process.
Actionable Insights
Customer analytics must yield actionable insights, not just passive, descriptive information. Examples of applying customer insights for downstream marketing actions include:
Analyze customer attributes (purchases, browse behavior, lifestyle interests) to determine next best actions for personalized marketing. Studies show personalized, relevant content consistently outperforms one-size-fits-all content by a large margin.
Isolate the distinctive traits of best customer segments to guide look-alike acquisition efforts. This leads to reduced outbound campaign costs and increased longer-term customer profitability of targeted customers.
Collect and enhance available data about recently acquired customers to predict customer lifetime value (CLV). Use the CLV measure to guide future development and retention efforts. Adjust the CLV score for each customer as new data points emerge.
Call to Action
Be obsessive about building and applying customer analytics to expose opportunities along the full customer experience journey.
Determine who is responsible for defining and building full lifecycle customer analytics.
Start with the customer data you have on hand. As mentioned above, it’s important to fill out the 360-degree view of your customers. But it’s more important to organize your existing customer data and start building the muscle for modeling, applying, and learning from customer analytics.
Create a roadmap for building more robust customer analytics. Start with a customer journey map and an inventory of customer data sources. Create a prioritized list of marketing use cases (personalization, look-alike targeting, surprise and delight, pre-emptive retention). Then connect the dots to determine what additional customer data sources are required. Investigate the potential for a marketing-managed CDP to ingest, match, and score your customer data. Build the business case. Go.
If your company is wondering how to put these application points into practice, feel free reach out to us at marketing@credera.com. We’d love to help as you think through how your company can capitalize on this new era of customer analytics.
Contact Us
Ready to achieve your vision? We're here to help.
We'd love to start a conversation. Fill out the form and we'll connect you with the right person.
Searching for a new career?
View job openings