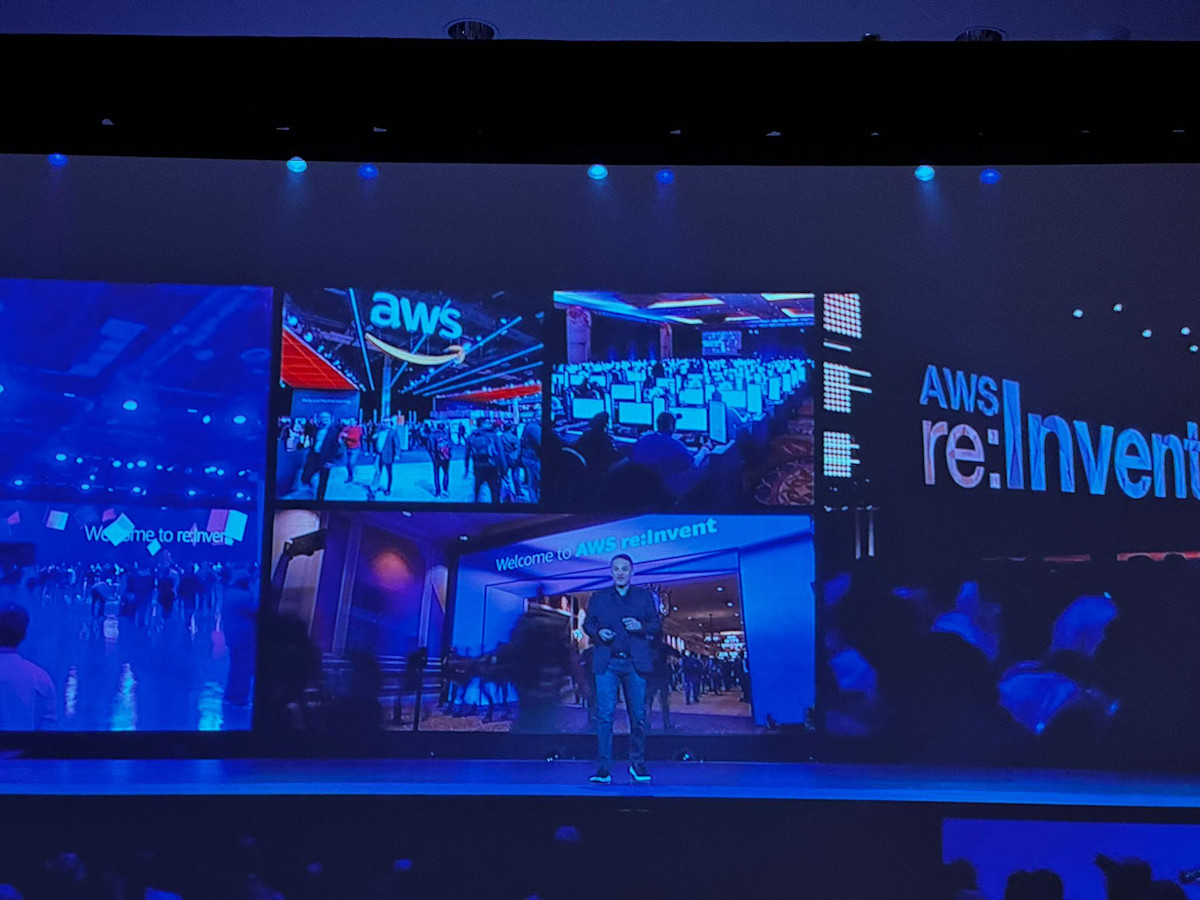
Credera attended the 11th annual AWS re:Invent conference in Las Vegas this year —an event that was attended live by over 50,000 people. In our previous article, we summarized some of the key takeaways from Adam Selipsky’s keynote speech.
In this article, Managing Consultant and data expert Naveen Swaminathan looks at some of the key announcements made at this year’s conference through a data lens and explores what new product features and enhancements will mean for businesses.
Data Analytics
Amazon DataZone Amazon previewed a new data management service that makes it easier for data producers to manage and govern access to data, enabling consumers to discover, use, and collaborate on data-driven business insights.
Amazon said, “Amazon DataZone removes the heavy lifting of maintaining a catalogue by using machine learning to collect and suggest metadata (e.g., origin and data type) for each dataset and by training on a customer’s taxonomy and preferences to improve over time.”
Amazon DataZone immediately reminded me of tools such as Collibra or IBM Cloud Pak for Data, which both offer similar capabilities. However, the key differentiator is the integration for DataZone within AWS ecosystem services such as Redshift, Athena, SageMaker, QuickSight, and Glue Catalogue. This product is perhaps long overdue in AWS’s arsenal and could be a fundamental building block for future data strategies.
Amazon previewed AWS Clean Room, a new service that makes it easier for customers and their partners to analyze and collaborate on their collective datasets and gain new insights without revealing underlying data. It is particularly useful for optimizing marketing and advertising experiences by providing enhanced customer insights.
Unlike other clean room offerings in the market, AWS Clean Room is different, particularly in cryptographic computing (C3R). Amazon claims that the service “allows you to collaborate using secure multi-party computation (SMPC)—a technique that allows multiple parties to jointly compute a function over their inputs while keeping those inputs private. SMPC ensures that data used in collaborative computations remains encrypted: at rest, in transit, and in use.”
It is worth noting that this service doesn’t extend to AWS taking responsibility for data security (encryption) and data privacy (GDPR/PII). These will need to be carefully considered as part of each organization's design phases.
The company announced a new feature in Amazon QuickSight Q that enabled forecasting and the ability to ask “why” questions. It uses machine learning (ML) and natural language processing to answer customer questions. Automated data preparation makes it faster for customers to start asking questions of their data using Amazon QuickSight Q. I would consider this a handy upgrade to an already good service.
Amazon revealed how AWS Glue Data Quality reduces time spent on data analysis and rule identification from days to hours by automatically measuring, monitoring, and managing data quality in data lakes and across data pipelines.
Over time, organizations have built data lakes and are continuing to build them. Without data quality, my view is that those data lakes soon become “data swamps,” which prevents effective analytics. AWS Glue Data Quality is expected to fill in this gap and it will be a handy tool for data architects to maintain quality of the data lake.
Amazon Redshift Integration for Apache Spark
Amazon Redshift integration for Apache Spark makes it easier for customers to run Apache Spark applications on data from Amazon Redshift using AWS analytics and machine learning services. In the keynote session, Amazon claims, “Amazon Redshift Integration for Apache spark is 10 times faster, and with optimized spark runtime and performance at scale, developers can begin running queries on Amazon Redshift data from Apache Spark-based applications within seconds using popular language frameworks (e.g., Python, R, and Scala).”
We have seen clients who are reliant on third-party and open-source connectors work around this limitation, so this service would be a particularly interesting proposition for them. It is needed to integrate with AWS’ most popular services and I intend to adopt it in my future engagements where the use case supports. As always, the devil is in the detail, and this integration may only work in the latest version of the services (for example, EMR 6.9 spark driver onward).
Amazon has now added a new feature to its Athena Engine for Apache Spark, which enables customers to get started with interactive analytics using Apache Spark in less than a second.
Apache Spark is a widely adopted processing engine for complex data analysis. Querying data and using various Amazon services without the need for any expensive resources will be largely welcomed in the data analytics and exploration community.
Amazon Aurora Zero-ETL Integration with Amazon Redshift
Amazon Aurora zero-ETL integration with Amazon Redshift enables customers to analyze petabytes of transactional data in near real-time, eliminating the need for custom data pipelines. AWS claims that building and maintaining ETL jobs are painful and expensive, so AWS set its vision for a “zero ETL future” to make life easier for their customers.
Azure also has a similar capability between Azure CosmosDB and Azure Synapsys. I’m excited to see the real-time insights and analytics capabilities that are possible with this new service.
Serverless
The rise of event-driven architectures has paved the way for more features to be added to existing event services within AWS. Amazon has now introduced Amazon EventBridge Pipes, which allow users to easily stitch AWS services together. EventBridge Pipes may have its use cases where suitable, but I believe this provides yet another choice for event-driven architecture on AWS alongside SQS, SNS, and EventBridge—a space that is already overloaded.
Machine Learning & Artificial Intelligence
Amazon has announced a range of new feature enhancements to improve SageMaker - Amazon’s machine learning service. Along with a newly redesigned user interface for SageMaker studio, I have listed some of these new enhancements below:
Amazon SageMaker Role Manager: Makes it easier for administrators to control access and define permissions for improved machine learning governance.
Amazon SageMaker Model Cards: To document and review model information throughout the machine learning lifecycle.
Amazon SageMaker Model Dashboard: Provides a central interface to track models, monitor performance, and review historical behavior.
Amazon SageMaker Studio Notebooks: The company announced a data preparation capability in Studio Notebook to help customers visually inspect and address data-quality issues in a few clicks. It also adds real-time collaboration for data science teams within Amazon SageMaker Studio Notebook and converts notebook code into production-ready jobs.
At Credera, we have undertaken and implemented machine learning infrastructure with fully compliant MLOps frameworks using Amazon SageMaker. AWS has addressed some of the shortcomings during our implementation in the model governance and collaboration space. As SageMaker is integrated into more and more AWS services for data, it will become widely adopted by organizations for advanced analytics.
Security
Amazon previewed Amazon Security Lake - a service that automatically centralizes an organizations's security data from cloud and on-premises sources into a purpose-built data lake in a customer’s AWS account. This allows customers to act on data security faster. Acknowledging that data has become the lifeblood of enterprises, Amazon also announced several updates to its data security services such as Amazon GuardDuty RDS Protection and Amazon Verified Permissions.
Compute Updates
Amazon shared a major update to its high-performance computing (HPC) offering by boosting updates on Hpc6id instances, a new chipset called Graviton3E for HPC, next generation Nitro smart networking chip, plus instances to its existing arsenal of processors in constant search for performance in its EC2 fleet.
I’ll certainly have to check out some of the HPC processors [AWS Graviton] with Amazon SageMaker to take advantage of the price, performance, and efficiency benefits that comes with Graviton chips.
What is one thing leaders should take away from AWS re:Invent 2022?
For me, it’s “Amazon DataZone.” In my own experience, data silos, data governance, and data sharing are all major problems facing organizations of today who are working to build a future-proof foundation. While there are some real architectural considerations to be made with DataZone, its successful implementation could just make a big difference.
As an Advanced Consulting Partner, Credera offers our clients end-to-end AWS solutions that increase flexibility, scalability, and security while helping them innovate and improve performance.
We have expertise in delivering AWS projects for DevOps, machine learning, security, migration, and big data. Leveraging our capabilities in marketing and commerce, digital media, and financial services, we help organizations solve immediate and long-term challenges by leveraging native services from AWS.
If you’d like to learn more, reach out to us at marketing@credera.com.
Contact Us
Ready to achieve your vision? We're here to help.
We'd love to start a conversation. Fill out the form and we'll connect you with the right person.
Searching for a new career?
View job openings