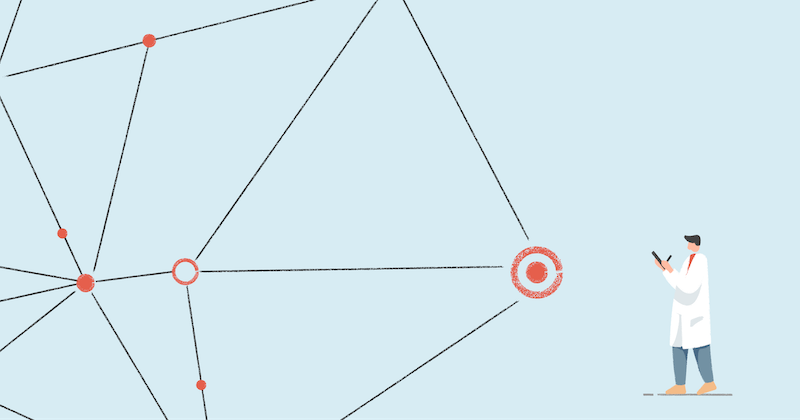
In this four-part series, we offer practical advice on how organisations can successfully realise value from data by running through the detail of our eight-layer data operating model.
In this third instalment, we address the fifth and sixth layers: ‘Partners & Locations' and ‘Process.' We will provide an overview of the challenges faced in these areas and explain the important factors to consider when designing and implementing your data operating model.
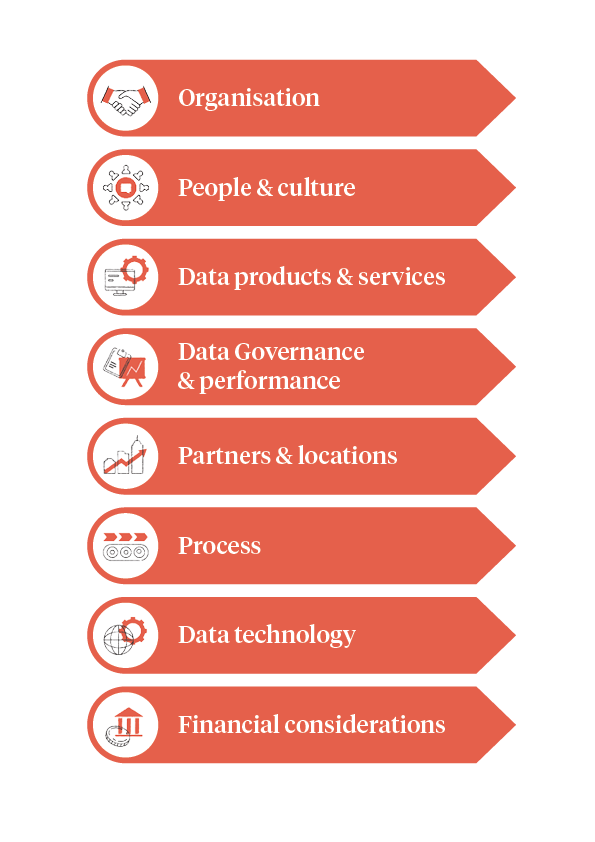
Read next: Whitepaper: Redefining Data Governance: How to let data govern your organisation
Partners & Locations
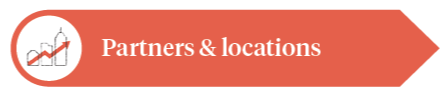
It is rare for an organisation to find all of their knowledge and capabilities solely within their company walls. More often than not, data organisations use a combination of internal knowledge and processes alongside external partners that may ultimately deliver data sources, data management rules, data processing, and more. Data transformations are best delivered with a combination of internal knowledge alongside experienced external change specialists to guide the process.
Who an organisation chooses to align with for ongoing data support is incredibly important as it will shape the possibilities available to them as well as the ability to scale in the future. The organisation should therefore consider whether it will purchase COTS (Commercial Off-the-Shelf) packages or a tailored bespoke tool to manage areas such as data quality, data lineage, data management, or data warehousing, amongst others. These decisions will form the basis of an organisation’s data guiding principles (similar to architectural guiding principles when deciding on a new suite of applications or platform) to ensure that all partners are able to work collaboratively together where required.
In a similar fashion, how an organisation splits investment between itself and third parties will help to balance the risk taken across all parties involved. This will be reflected in the data strategy roadmap helping to ensure that both the organisation and partners are aware of timelines, budgets, and delivery expectations, whilst also making new partners aware of the operating conditions as they are introduced into the journey. A shared commitment needs to be encouraged as the organisation embarks on its data transformation journey.
Partners involved in data processing could also include those that supply third party data. Commonly used third party data sets include reference address data from suppliers such as Royal Mail. When ingesting external data, the purchaser needs to consider how they can assess the quality, any transformation requirements (e.g. date and time formats), and any agreements / contractual obligations with the data supplier.
Process

A critical section of the data operating model considers how processes and technology will work together to enable efficiency, drive alignment, and produce metrics to support decision making and ensure successful data operations. These processes can be either manual or automated. As the latter frees up employees to focus on more business critical and value driven tasks, it is what many organisations strive to achieve. Examples of data processes include:
Data access
By having a clear and up-to-date record of where data is mastered, stored, and located, the organisation can deploy role-based access control (RBAC) models or similar to ensure that data is freely available only to those who need it. As part of the process, there should be clear communications with the business as to how an individual or team can allocate the best data possible to allow them to be successful in their data activities.
Data governance processes
There are a number of data governance processes that need to be considered, but most of them focus on clear routes to share metrics at different levels of the organisation (e.g. either for a specific region, for a certain team, or rolled up to an executive committee). The metrics can include aspects such as key users of the data (data access metrics), which data sets are prominently used (data usage metrics) and data quality metrics. Each of these metrics should guide the specific area of the business in making clear decisions on how to manage and use their captured data. Alongside metrics, data governance processes should include instructions or procedures on how escalations, committees or forums, and data fixes (including who is required to resolve them) should operate.
Data quality improvement
The data an organisation uses for processing and the decision making from analytics is only as good as the quality of the data. To ensure that the captured data is of high quality, the organisation needs to first be able to measure it. Having a set of enterprise-level rules deployed to a golden source within each domain will ensure consistency across the organisation. This might include a consistent rule to assess the accuracy of a date is applied in the same way in both a customer and product data set. The data quality metrics will often have coverage across all of the data quality dimensions (i.e. accuracy, timeliness, completeness, consistency, uniqueness etc.). These metrics are best displayed in dashboards and will provide two benefits. Firstly, it will allow the identification of the golden record (or best version of the data) and support the data engineers in their resolution management. Secondly, it enables the roll up of metrics to deliver the right information for the level that it is being presented at – whether that be team, domain, or executive.
Classifying data sets
Within each data domain (division of data across the organisation), the use of metadata will enable data sets to be classified. This will support in the identification and selection of the best data set to be used by data consumers. It also reduces data duplication, saving money on storage, and helping to ensure that data is GDPR compliant as a result.
Data analytics and data visualisation
The majority of organisations recognise the importance of data analytics to help an organisation to make clear business decisions and drive a competitive advantage. We have touched upon the foundations of having good data analytics to ensure positive data quality, classifying data sets, and having clear metrics in place. Once these foundations are in place, the enterprise can begin to look into having self-serving tooling patterns and templates to enable data discovery and analytics. This can provide several benefits including consistent outputs being established across the enterprise; the data consumer being directed to the golden sources; role-based access requests enabling individuals to only see the data that matches their privileges; and time saved by having portals which hold the relevant information.
Data virtualisation, master data management, and ETL technologies
How a company controls and manages their data is of critical day-to-day importance. Ensuring that data is mastered correctly prevents data repetition, clashes, and inaccuracies as it is used around the organisation. Having been around for several years and being well established within infrastructure, ETL (export, transport, and load) processes and data virtualisation are likely to be the most well known data processes. Today, most of these processes are highly automated due to consistencies in where data products are known for how long they will take to process, the amount of computing power, and the technology that they travel across.
Data matching and single customer view
As organisations have grown and developed new product or service lines, been acquired, merged, or acquired other firms, new platforms and processes are introduced. This often leads to the organisation having multiple views of the customer depending on which system is accessed. Whilst all of this data could be deemed as correct and not necessarily picked up by data quality rules, the impact is reduced insight through analytics. Being able to conclusively state whether data entries are connected uses a method called data matching and often uses flawed logic to give a confidence rating on whether individuals from disparate systems are in fact the same. This method boosts the information behind the customer (e.g. latest telephone number or address) or even how that customer interacts with the company (e.g. through social media, products bought, or use of a loyalty card, etc).
Data lineage
Understanding where data is created, mastered, and stored across the enterprise will ensure that the business can implement effective archiving, retention, and deletion protocols. Having these in place will ensure regulatory compliance and also that data is used where there is a legitimate interest and not kept longer than required. Further to this, organisations are exploring automated data lineage using a variety of the latest techniques and tools to ensure that the knowledge of where data is at any given time is up-to-date.
Data security and regulatory compliance
Finding the balance between ensuring that data is protected and secured whilst enabling access to specific people within an organisation can be a tricky balance. Assessing and tagging data at a logical level and then at a physical systems level (containing personal identifiable information (PII) and special personal identifiable information (SPII) will enable an organisation to ensure extra protections are made. These protections will apply to those who have access to the data sets, and also apply additional encryptions in place where data is stored, accessed, and transported.
In a nutshell
Who an organisation chooses to align with for ongoing data support is incredibly important as it will shape the possibilities available to them as well as the ability to scale in future. How an organisation splits investment between itself and third parties will help to balance any risk taken across all parties involved. Robust and coherent data-specific processes form an essential part of how an organisation and its partners operate, helping to mitigate risk and maximise value. In our next article, we address layers seven and eight: ‘Data Technology’ and ‘Financial Consideration’.
Contact Us
Ready to achieve your vision? We're here to help.
We'd love to start a conversation. Fill out the form and we'll connect you with the right person.
Searching for a new career?
View job openings