AI
Apr 30, 2024
Barriers to Gen AI adoption and how AWS can help: Key takeaways from the AWS London Summit
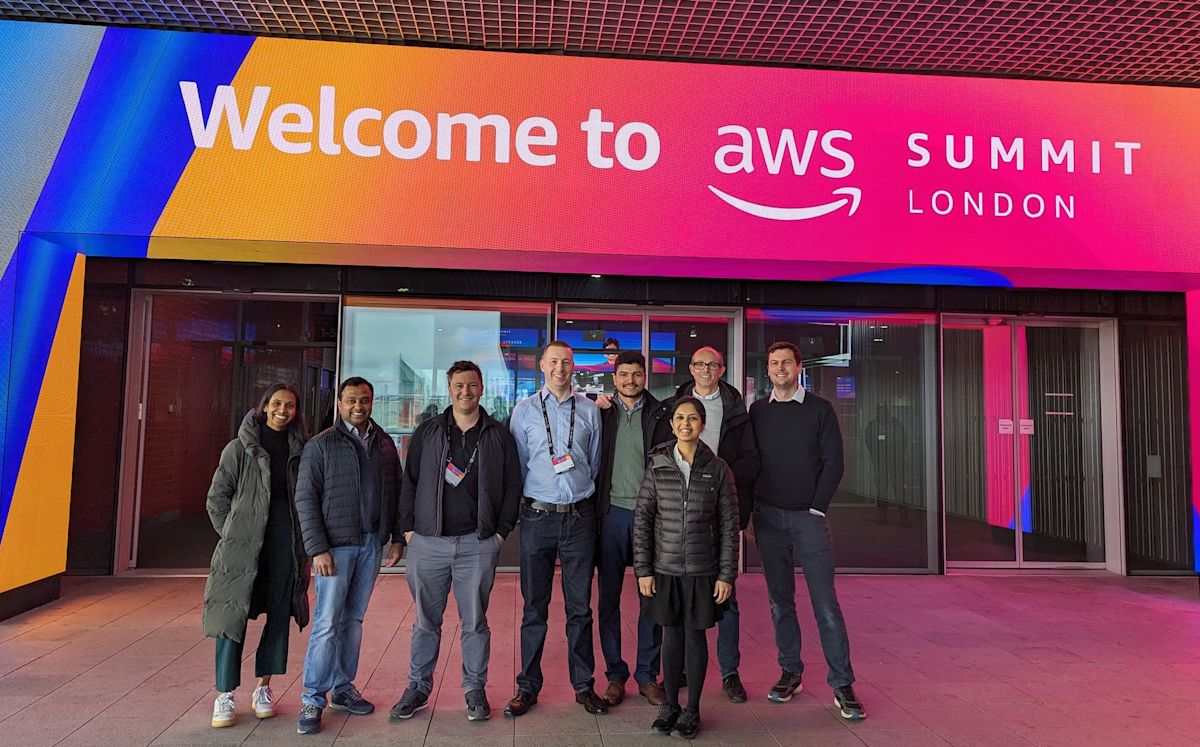
In April 2024, Credera attended the AWS Summit in London to explore the frontiers of cloud technology and innovation. In this blog, we reflect on some of the key takeaways from this year’s event, including some of the main pain points experienced by customers starting out on their Gen AI journey and how AWS can help customers overcome these challenges.
The potential of Generative AI
It probably doesn’t come as a surprise that one of the key themes to emerge from the 2024 AWS London Summit was Generative AI. Since the release of ChatGPT, organisations have been racing to understand the opportunity that Gen AI holds for them and how they can start unlocking it’s potential. As with any new technology, however, full scale adoption doesn’t happen overnight. It takes time to build the right foundations, teams, and processes before you can really start to see the returns.
At the Summit, AWS kicked off with a keynote that touched on the latest tools and services it offers to help customers accelerate their adoption of Gen AI. AWS customers, including Zilch and TUI, spoke about how they have leveraged these services and aim to use them in future to supercharge their capabilities. AWS’s Francessca Vasquez also acknowledged the challenges that customers have faced with Gen AI adoption over the last year and pointed to AWS’s generative AI stack to help customers overcome some of these difficulties and start realising the potential of AI.
Challenges to Gen AI adoption
Whilst there has been a lot of excitement around Gen AI recently, businesses have faced a myriad of obstacles when trying to experiment with the technology and understand how it will transform the way they work. These challenges relate to every aspect of the large language models (LLMs) that form the basis of Gen AI: the hardware LLMs run on, the data they are trained on, and the accuracy of their responses. Below are some of the biggest obstacles facing Gen AI adoption:
1. Data strategy is not fit for purpose
This is a big one. “Data is your differentiator” and “Good data = Good AI” are some of the phrases that were echoed throughout the Summit. It’s clear that in order to get the most out of Gen AI, businesses will need to have a clearly defined and robust data strategy. However, for many organisations, this is still a long way away.
First, there is the quality of data. LLMs are trained on vast amounts of data and the quality of this data will directly determine how the final model will perform. Even a small discrepancy can have a significant impact on the accuracy of the final model. Gathering good quality data requires a joined-up approach across multiple teams to discover, integrate, categorise, and process data, none of which are straightforward tasks.
The quality of data will be underpinned by having good governance and standards in place to ensure data is being collected and used in the right way throughout its lifecycle.
The way data is stored and retrieved is another key consideration due to the vast amounts of data required to train models, as well as storing responses from models to be used elsewhere.
One of the biggest issues users have faced with LLMs is hallucination, which is where the model returns a response that is factually inaccurate or nonsensical. This can often be attributed to a lack of complete, accurate, and relevant data that leads to gaps in the model’s understanding.
2. Lack of expertise
Whilst many businesses are expecting to use AI-related tools in the next few years, many of them highlight the fact that they are struggling to find the AI talent they need to start this journey. Furthermore, developments in Gen AI are still moving at lightening pace and keeping up with this is proving to be challenging, even for those working directly with AI.
3. Computational constraints
LLMs require significant Enterprise Class Graphics Processing Unit (GPU) resources both for training and when deployed. GPUs are neither cheap nor without significant lead times, and the high cost makes it difficult to scale as businesses grow.
4. Integrating with existing systems
To get the most out of AI, businesses will look to integrate Gen AI capabilities into existing systems and processes which can often require a considerable amount of work.
5. Cyber security threats
As with any new technology, increased capabilities can often mean increased threats. This includes injection or poisoning attacks to corrupt models or the responses they return, extracting sensitive data from models and DDoS attacks to reduce availability.
6. High energy demand
We have already discussed the huge computational requirements of AI models and it’s no secret that data centres have huge energy demands. As we collectively move towards being more sustainable and businesses aim to meet their suitability goals, the energy requirements related to Gen AI use are becoming a problem that requires a quick solution.
7. Not knowing where to start
The vast amounts of information about Gen AI and its potential uses can be overwhelming. Businesses are keen to adopt the technology but may struggle with knowing where to start. For example, there are now a huge range of LLM models to choose from, all with a broad set of capabilities. Businesses need greater insight on which model to use for different use cases.
How can AWS help?
Throughout the Summit, AWS showcased the various services and tools it offers to help customers overcome some of the challenges associated with Gen AI and make it more accessible to all. The AWS Generative AI tech stack was highlighted in the keynote and throughout the Summit and encapsulates many of these key services in each layer:
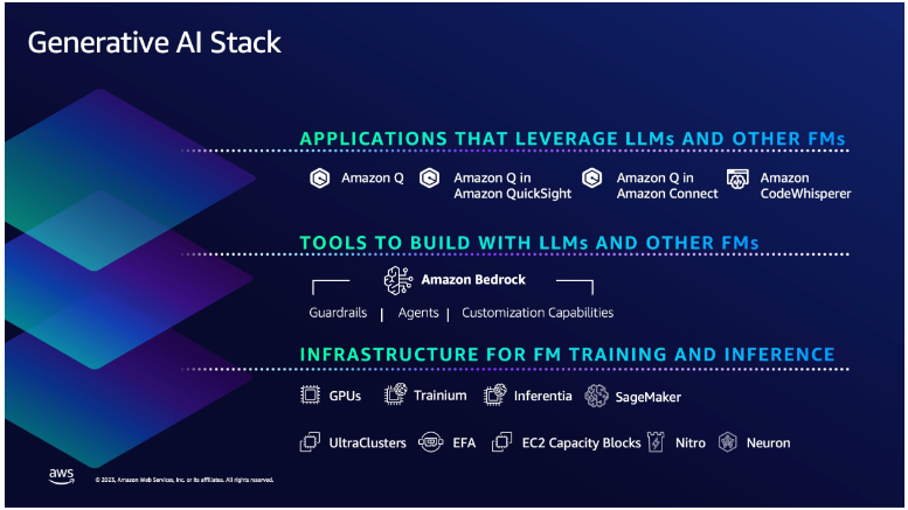
Amazon SageMaker
Amazon SageMaker is a service that has been around for a few years and is a
machine learning platform that allows the creation, training, and deployment of machine learning models. There have been recent additions to this service that offer customers the ability to overcome some of the barriers to AI adoption:
SageMaker Data Wrangler offers a fast and easy way to prepare unstructured data for use with AI models. Data can be selected from various sources via SQL and be imported quickly. It also provides a data quality and insights report to automatically verify data quality and detect anomalies.
Amazon SageMaker Canvas provides a visual no code interface through which customers can prepare data for use with AI models. This tool can be used with no machine learning experience and doesn’t require any code to be written.
Amazon SageMaker HyperPod provides purpose-built infrastructure for distributed model training at scale.
Amazon Bedrock
AWS announced that one of its most exciting new services, Amazon Bedrock, is coming to London. Amazon Bedrock is a fully managed service that offers a range of foundation models that can be used to build and scale Generative AI applications securely.
It offers a platform to experiment with different foundation models, such as Claude and Llama, and identify which one might be best for a specific use case. The models can be privately customised to your business by using data to fine-tune models and Retrieval Augmented Generation (RAG) to provide it with the latest data, therefore reducing occurrences of hallucination. It also offers the ability to build agents that applications can run to execute tasks on your systems and data sources, making integration with your other systems easier.
The data you use to fine-tune models will remain under your control. It’s not stored or logged by Amazon or used to train the underlying foundational model.
To prevent unwanted content moving between the model and applications, guardrails can be used that sit between a model and an application and restrict what can pass between them.
As the service is serverless, users don’t need to provision and manage any of the underlying infrastructure, making the high computational requirements less of a barrier to entry.
Amazon Q
Amazon Q is another relatively new service that has huge potential for those looking to adopt Gen AI. It is a Gen AI-powered assistant that can be tailored to your business and used across AWS in a huge variety of different use cases.
There are several Amazon Q products that AWS offer, two of which are Amazon Q Developer and Business:
Amazon Q Developer: Amazon Q has been trained on 17 years of AWS documentation, which means it is able to provide useful insight and recommendations to customers looking to architect, build, test, and deploy solutions on AWS following best practices. The insight can increase productivity and free up time for technical resources, as well as aiding less experienced AWS users in building the knowledge more quickly.
Amazon Q Business: Amazon Q can be trained on data specific to your business which then enables it to answer questions, generate content, and complete tasks specific to you. It offers 40+ connectors to popular enterprise applications and document repositories, enabling it to bring together disparate data. It has also been built to understand and comply with existing identities, roles, and permissions in Identity and Access Management (IAM).
Amazon DataZone
To support customers with managing vast amounts of data across their organisations, AWS provides Amazon DataZone. This data management service can aid customers in discovering, cataloguing, and sharing data stored across AWS regions, on premise, and third-party sources. It also enables better governance of data across an organisation, ensuring that the right users can access the right data based on their roles and permissions.
Sustainability pillar
AWS have recently added a new Sustainability pillar to their AWS Well-Architected Framework, which provides design principles, operational guidance, and best practices to help customers meet their sustainability goals. Some of the recommendations outlined include implementing data management practices to move more data to energy efficient storage tiers and review architecture to consolidate under-utilised components.
In summary
Whilst organisations are keen to tap into the potential of Gen AI, there are significant obstacles that can prevent large-scale adoption or even just the process of getting started. Some of these challenges have long been issues for businesses but they are now more important than ever. This includes having the right data strategy and foundations in place, acquiring and developing the right expertise, and integrating disparate systems.
Over the last few months, AWS have announced a number of services and tools that can support customers in overcoming these obstacles and help them move forward in their Gen AI adoption journeys. Whilst these services are a great enabler, the key will also be knowing how to use them in the right way to fully harness the potential of Gen AI for your organisation.
How we can help
As a Premier Consulting Partner, we strive to make an extraordinary impact for our clients by solving their business challenges whilst harnessing the power of AWS services to simplify, modernise, and scale solutions at pace. We have first-class expertise in AI/ML, MLOps, Data Analytics, and DevOps, and using our extensive industry knowledge, we aim to help our clients to continue to gain a competitive advantage.
Download our generative AI for CIOs whitepaper
Customer Experience Transformation Worksheet
Contact Us
Ready to achieve your vision? We're here to help.
We'd love to start a conversation. Fill out the form and we'll connect you with the right person.
Searching for a new career?
View job openings